AI is Changing Everything We Thought About Forecasting Demand
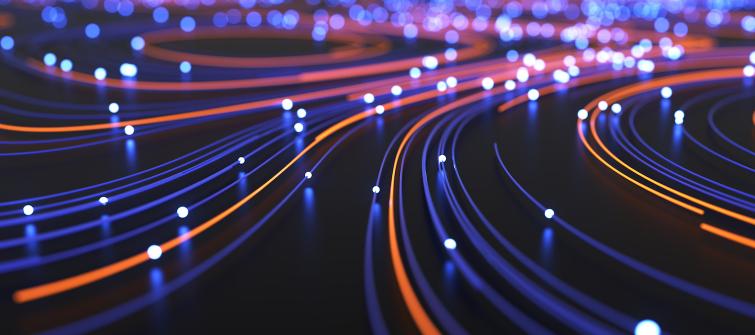
The rise of cloud computing, smart devices, and IoT have all resulted in a massive data explosion. IDC estimates that by 2025, digital data created worldwide will rise to 175 zettabytes (from approximately 40 zettabytes in 2019). AI now has the ability to consume data far more efficiently than humans, delivering on the possibility of insights and predictions never before imagined. McKinsey Global Institute states that data and AI are not only finding answers faster but creating transparency around issues that have always been murky.
But predicting future demand with traditional methods in the face of a shifting market that is constantly experiencing disruption can become a serious barrier to visibility into what lies ahead. Many sources reinforce that the number one obstacle to achieving supply chain planning goals and objectives continues to be forecast accuracy and demand variability. This trend continues despite the investment many have made in demand planning technology designed to better predict customer demand.
Why does this challenge continue to be so prevalent? One of Coupa’s demand solution customers, Donald Anderson, Assistant Director of Analysis, Air Mobility Command – U.S. Air Force, made a very poignant statement, “Our past methodology was time-series trend forecasting. This works great … until the world changes.”
This is then the crux of the issue for many. Traditional time-series demand forecasting typically means taking an inside-out approach, often relying on an organization’s historical, internal data to predict demand. The danger is it doesn’t take into account external factors that continually impact the market. Change is accelerating faster than inside the four walls of today’s enterprises. Data is growing exponentially, technology continues to disrupt, customers demand an omni channel buying experience and businesses are constantly grappling with macroeconomic conditions and industry complexities. While historical performance may offer helpful insights toward what the future holds, change is happening far too rapidly to rely on internal rear-view mirror metrics.
Effectively responding to complexity and disruption is increasingly dependent on organizations’ ability to absorb data — internal and external — and process the data into intelligent insights driving decisions in a timely manner. In the world of data and AI, most organizations are operating with a mixed bag of legacy technologies into which they invested millions of dollars that they simply can’t turn off. However, they are forced to compete in their respective industries with the digital natives that are building business models completely embracing the power of data and algorithms.
How can organizations harness the promise of powerful insights for better demand predictions with data and technology? Enter the practical adoption of digital transformation via platforms, applications and predictive models, fed by a foundational data layer, powered by AI and enabled with real-world scenario planning.
1. AI-Powered Pattern Recognition
Here, AI is applied to a company’s historical demand signal to demystify it in an unbiased way. The technology self-learns from complex demand patterns (e.g., multiple seasonality signals, non-linear trends, lags, level shifts, etc.) and finds common groups for segmentation to prescribe the appropriate model. AI-powered demand models are essentially closed loop systems. As they drive decisions, they monitor and adapt from changes in the data.
2. Internal and External Causals
Once complex patterns from historical data are identified, the next step is to bring in the effects of internal and external causal factors to enhance the demand signal and quantify key demand drivers. Internal variables may include price, promotions, product lifecycles and point-of-sale data. There’s a wealth of external data that can be incorporated, such as macroeconomic indicators like GDP and Consumer Price Index, regional weather data, market share data, consumer sentiment, demographic trends and much more.
3. What-If Scenarios
The ability to model what-if scenarios for more accurate predictions is the lynchpin to next-generation demand modeling. This is the pivotal point where organizations can conquer cause and effect by testing demand scenarios and sensitivities at varying levels of granularity. Factoring these scenarios into demand models with greater depth and predictiveness prepares decision makers for changing conditions.
4. Near-Term Probabilistic Forecasting
AI can also play a strong role in discerning patterns and delivering insights for short term forecasts. Predicting actual orders using data like page-views and current inventory positions from vendor portals, companies can set up recommendations on inventory positioning by weighing the tradeoff of stockouts vs excess inventory, also significantly improving service levels.
Organizations are seeing that they can reinvent how they forecast with the latest AI technologies. Cloud computing is bringing scale to forecasting. AutoML (automated machine learning) helps build more effective models. Hierarchical forecasting allows for better reconciled top-down and bottom up forecasting. And, algorithms that take advantage of deep learning are delivering improvements through cross-learning, using internal and external data inputs to detect patterns. The resulting insights provide organizations with the ability to adapt to shifting external dynamics and accelerate enterprise-wide decisions about supply chain scalability, sourcing strategies, product portfolio roadmaps, and capacity planning can mean the difference between competitive dominance and obsolescence.
With never normal being the new normal, now is the time for market leaders to take an AI-powered, outside-in approach to predicting demand.